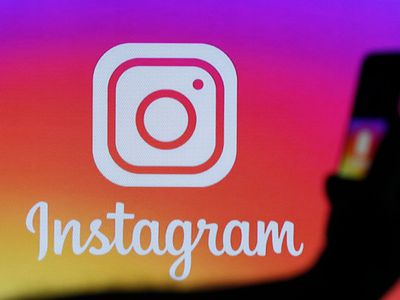
Preventing Oversharing: Secure Neural Networks

Preventing Oversharing: Secure Neural Networks
Disclaimer: This post includes affiliate links
If you click on a link and make a purchase, I may receive a commission at no extra cost to you.
Key Takeaways
- Neural network model inversion attacks use AI chatbots to uncover and reconstruct personal information from digital footprints.
- Hackers create inversion models that predict the inputs based on the outputs of a neural network, revealing sensitive data.
- Techniques like differential privacy, multi-party computation, and federated learning can help protect against inversion attacks, but it’s a continuous battle. Users should be selective sharers, keep software updated, and be cautious about providing personal information.
MUO VIDEO OF THE DAY
SCROLL TO CONTINUE WITH CONTENT
Imagine you’re at a restaurant and just tasted the best cake you’ve ever eaten. Back at your home, you’re determined to recreate this culinary masterpiece. Instead of asking for the recipe, you rely on your taste buds and knowledge to deconstruct the dessert and whip up your own.
Now, what if someone could do that with your personal information? Someone tastes the digital footprint you leave behind and reconstructs your private details.
That’s the essence of a neural network model inversion attack, a technique that could turn an AI chatbot into a cyber sleuthing tool.
Understanding Neural Network Model Inversion Attacks
A neural network is the “brain” behind modern artificial intelligence (AI). They’re responsible for the impressive functionality behind voice recognition, humanized chatbots, and generative AI.
Neural networks are essentially a series of algorithms designed to recognize patterns, think, and even learn like a human brain. They do so at a scale and speed that far surpasses our organic capabilities.
AI’s Book of Secrets
Just like our human brain, neural networks can hide secrets. These secrets are the data its users have fed them. In a model inversion attack, a hacker uses the outputs of a neural network (like the responses from a chatbot) to reverse-engineer the inputs (the information you’ve provided).
To execute the attack, hackers use their own machine learning model called an “inversion model.” This model is designed to be a mirror image of sorts, trained not on the original data but on the outputs generated by the target.
The purpose of this inversion model is to predict the inputs—the original, often sensitive data that you have fed into the chatbot.
Creating the Inversion Model
Creating the inversion can be thought of as reconstructing a shredded document. But instead of piecing together strips of paper, it’s piecing together the story told to the target model’s responses.
The inversion model learns the language of the neural network’s outputs. It looks for telltale signs that, with time, reveal the nature of the inputs. With each new piece of data and each response it analyzes, it better predicts the information you provide.
This process is a constant cycle of hypothesis and testing. With enough outputs, the inversion model can accurately infer a detailed profile of you, even from the most innocuous-seeming data.
The inversion model’s process is a game of connecting the dots. Each piece of data leaked through the interaction allows the model to form a profile, and with enough time, the profile it forms is unexpectedly detailed.
Eventually, insights into the user’s activities, preferences, and identity are revealed. Insights that were not meant to be disclosed or made public.
What Makes It Possible?
Within neural networks, each query and response is a data point. Skilled attackers deploy advanced statistical methods to analyze these data points and seek correlations and patterns imperceptible to human understanding.
Techniques such as regression analysis (examining the relationship between two variables) to predict the values of the input based on the outputs you receive.
Hackers use machine learning algorithms in their own inversion models to refine their predictions. They take the outputs from the chatbot and feed them into their algorithms to train them to approximate the inverse function of the target neural network.
In simplified terms, “inverse function” refers to how the hackers reverse the data flow from output to input. The goal of the attacker is to train their inversion models to perform the opposite task of the original neural network.
In essence, this is how they create a model that, given the output alone, tries to calculate what the input must have been.
How Inversion Attacks Can Be Used Against You
Imagine you’re using a popular online health assessment tool. You type in your symptoms, previous conditions, dietary habits, and even drug use to get some insight into your well-being.
That’s sensitive and personal information .
With an inversion attack targeting the AI system you’re using, a hacker might be able to take the general advice the chatbot gives you and use it to infer your private medical history. For example, a response from the chatbot might be something like this:
Antinuclear antibody (ANA) can be used to indicate the presence of autoimmune diseases such as Lupus.
The inversion model can predict that the target user was asking questions related to an autoimmune condition. With more information and more responses, the hackers can infer that the target has a serious health condition. Suddenly, the helpful online tool becomes a digital peephole into your personal health.
What Can Be Done About Inversion Attacks?
Can we build a fort around our personal data ? Well, it’s complicated. Developers of neural networks can make it tougher to carry out inversion model attacks by adding layers of security and obscuring how they operate. Here are some examples of techniques employed to protect users:
- Differential Privacy: This ensures that AI outputs are sufficiently “noisy” to mask individual data points. It’s a little like whispering in a crowd—your words are lost in the collective chatter of those around you.
- Multi-Party Computation: This technique is like a team working on a confidential project by sharing only the results of their individual tasks, not the sensitive details. It enables multiple systems to process data together without exposing individual user data to the network—or each other.
- Federated Learning: Involves training an AI across multiple devices, all while keeping individual user’s data local. It’s a little like a choir singing together; you can hear every voice, but no single voice can be isolated or identified.
While these solutions are largely effective, protecting against inversion attacks is a cat-and-mouse game. As defenses improve, so do the techniques to bypass them. The responsibility, then, falls on the companies and developers that collect and store our data, but there are ways you can protect yourself.
How to Protect Yourself Against Inversion Attacks
Image Credit:Mike MacKenzie/Flickr
Relatively speaking, neural networks and AI technologies are still in their infancy. Until the systems are foolproof, the onus is on the user to be the first line of defense when protecting your data .
Here are a few tips on how to lower the risk of becoming a victim of an inversion attack:
- Be a Selective Sharer: Treat your personal information like a secret family recipe. Be selective about who you share it with, especially when filling out forms online and interacting with chatbots. Question the necessity of every piece of data that is requested of you. If you wouldn’t share the information with a stranger, don’t share it with a chatbot.
- Keep Software Updated: Updates to front-end software, browsers, and even your operating system are designed to keep you safe . While developers are busy protecting the neural networks, you can also reduce the risk of data interception by regularly applying patches and updates.
- Keep Personal Information Personal: Whenever an application or chatbot requests personal details, pause and consider the intent. If the requested information seems irrelevant to the service provided, it probably is.
You wouldn’t provide sensitive information like health, finances, or identity to a new acquaintance just because they said they required it. Similarly, gauge what information is truly necessary for an application to function and opt out of sharing more.
Safeguarding Our Personal Information in the Age of AI
Our personal information is our most valuable asset. Guarding it requires vigilance, both in how we choose to share information and in developing security measures for the services we use.
Awareness of these threats and taking steps such as those outlined in this article contributes to a stronger defense against these seemingly invisible attack vectors.
Let’s commit to a future where our private information remains just that: private.
MUO VIDEO OF THE DAY
SCROLL TO CONTINUE WITH CONTENT
Imagine you’re at a restaurant and just tasted the best cake you’ve ever eaten. Back at your home, you’re determined to recreate this culinary masterpiece. Instead of asking for the recipe, you rely on your taste buds and knowledge to deconstruct the dessert and whip up your own.
Now, what if someone could do that with your personal information? Someone tastes the digital footprint you leave behind and reconstructs your private details.
That’s the essence of a neural network model inversion attack, a technique that could turn an AI chatbot into a cyber sleuthing tool.
Understanding Neural Network Model Inversion Attacks
A neural network is the “brain” behind modern artificial intelligence (AI). They’re responsible for the impressive functionality behind voice recognition, humanized chatbots, and generative AI.
Neural networks are essentially a series of algorithms designed to recognize patterns, think, and even learn like a human brain. They do so at a scale and speed that far surpasses our organic capabilities.
AI’s Book of Secrets
Just like our human brain, neural networks can hide secrets. These secrets are the data its users have fed them. In a model inversion attack, a hacker uses the outputs of a neural network (like the responses from a chatbot) to reverse-engineer the inputs (the information you’ve provided).
To execute the attack, hackers use their own machine learning model called an “inversion model.” This model is designed to be a mirror image of sorts, trained not on the original data but on the outputs generated by the target.
The purpose of this inversion model is to predict the inputs—the original, often sensitive data that you have fed into the chatbot.
Creating the Inversion Model
Creating the inversion can be thought of as reconstructing a shredded document. But instead of piecing together strips of paper, it’s piecing together the story told to the target model’s responses.
The inversion model learns the language of the neural network’s outputs. It looks for telltale signs that, with time, reveal the nature of the inputs. With each new piece of data and each response it analyzes, it better predicts the information you provide.
This process is a constant cycle of hypothesis and testing. With enough outputs, the inversion model can accurately infer a detailed profile of you, even from the most innocuous-seeming data.
The inversion model’s process is a game of connecting the dots. Each piece of data leaked through the interaction allows the model to form a profile, and with enough time, the profile it forms is unexpectedly detailed.
Eventually, insights into the user’s activities, preferences, and identity are revealed. Insights that were not meant to be disclosed or made public.
What Makes It Possible?
Within neural networks, each query and response is a data point. Skilled attackers deploy advanced statistical methods to analyze these data points and seek correlations and patterns imperceptible to human understanding.
Techniques such as regression analysis (examining the relationship between two variables) to predict the values of the input based on the outputs you receive.
Hackers use machine learning algorithms in their own inversion models to refine their predictions. They take the outputs from the chatbot and feed them into their algorithms to train them to approximate the inverse function of the target neural network.
In simplified terms, “inverse function” refers to how the hackers reverse the data flow from output to input. The goal of the attacker is to train their inversion models to perform the opposite task of the original neural network.
In essence, this is how they create a model that, given the output alone, tries to calculate what the input must have been.
How Inversion Attacks Can Be Used Against You
Imagine you’re using a popular online health assessment tool. You type in your symptoms, previous conditions, dietary habits, and even drug use to get some insight into your well-being.
That’s sensitive and personal information .
With an inversion attack targeting the AI system you’re using, a hacker might be able to take the general advice the chatbot gives you and use it to infer your private medical history. For example, a response from the chatbot might be something like this:
Antinuclear antibody (ANA) can be used to indicate the presence of autoimmune diseases such as Lupus.
The inversion model can predict that the target user was asking questions related to an autoimmune condition. With more information and more responses, the hackers can infer that the target has a serious health condition. Suddenly, the helpful online tool becomes a digital peephole into your personal health.
What Can Be Done About Inversion Attacks?
Can we build a fort around our personal data ? Well, it’s complicated. Developers of neural networks can make it tougher to carry out inversion model attacks by adding layers of security and obscuring how they operate. Here are some examples of techniques employed to protect users:
- Differential Privacy: This ensures that AI outputs are sufficiently “noisy” to mask individual data points. It’s a little like whispering in a crowd—your words are lost in the collective chatter of those around you.
- Multi-Party Computation: This technique is like a team working on a confidential project by sharing only the results of their individual tasks, not the sensitive details. It enables multiple systems to process data together without exposing individual user data to the network—or each other.
- Federated Learning: Involves training an AI across multiple devices, all while keeping individual user’s data local. It’s a little like a choir singing together; you can hear every voice, but no single voice can be isolated or identified.
While these solutions are largely effective, protecting against inversion attacks is a cat-and-mouse game. As defenses improve, so do the techniques to bypass them. The responsibility, then, falls on the companies and developers that collect and store our data, but there are ways you can protect yourself.
How to Protect Yourself Against Inversion Attacks
Image Credit:Mike MacKenzie/Flickr
Relatively speaking, neural networks and AI technologies are still in their infancy. Until the systems are foolproof, the onus is on the user to be the first line of defense when protecting your data .
Here are a few tips on how to lower the risk of becoming a victim of an inversion attack:
- Be a Selective Sharer: Treat your personal information like a secret family recipe. Be selective about who you share it with, especially when filling out forms online and interacting with chatbots. Question the necessity of every piece of data that is requested of you. If you wouldn’t share the information with a stranger, don’t share it with a chatbot.
- Keep Software Updated: Updates to front-end software, browsers, and even your operating system are designed to keep you safe . While developers are busy protecting the neural networks, you can also reduce the risk of data interception by regularly applying patches and updates.
- Keep Personal Information Personal: Whenever an application or chatbot requests personal details, pause and consider the intent. If the requested information seems irrelevant to the service provided, it probably is.
You wouldn’t provide sensitive information like health, finances, or identity to a new acquaintance just because they said they required it. Similarly, gauge what information is truly necessary for an application to function and opt out of sharing more.
Safeguarding Our Personal Information in the Age of AI
Our personal information is our most valuable asset. Guarding it requires vigilance, both in how we choose to share information and in developing security measures for the services we use.
Awareness of these threats and taking steps such as those outlined in this article contributes to a stronger defense against these seemingly invisible attack vectors.
Let’s commit to a future where our private information remains just that: private.
MUO VIDEO OF THE DAY
SCROLL TO CONTINUE WITH CONTENT
Imagine you’re at a restaurant and just tasted the best cake you’ve ever eaten. Back at your home, you’re determined to recreate this culinary masterpiece. Instead of asking for the recipe, you rely on your taste buds and knowledge to deconstruct the dessert and whip up your own.
Now, what if someone could do that with your personal information? Someone tastes the digital footprint you leave behind and reconstructs your private details.
That’s the essence of a neural network model inversion attack, a technique that could turn an AI chatbot into a cyber sleuthing tool.
Understanding Neural Network Model Inversion Attacks
A neural network is the “brain” behind modern artificial intelligence (AI). They’re responsible for the impressive functionality behind voice recognition, humanized chatbots, and generative AI.
Neural networks are essentially a series of algorithms designed to recognize patterns, think, and even learn like a human brain. They do so at a scale and speed that far surpasses our organic capabilities.
AI’s Book of Secrets
Just like our human brain, neural networks can hide secrets. These secrets are the data its users have fed them. In a model inversion attack, a hacker uses the outputs of a neural network (like the responses from a chatbot) to reverse-engineer the inputs (the information you’ve provided).
To execute the attack, hackers use their own machine learning model called an “inversion model.” This model is designed to be a mirror image of sorts, trained not on the original data but on the outputs generated by the target.
The purpose of this inversion model is to predict the inputs—the original, often sensitive data that you have fed into the chatbot.
Creating the Inversion Model
Creating the inversion can be thought of as reconstructing a shredded document. But instead of piecing together strips of paper, it’s piecing together the story told to the target model’s responses.
The inversion model learns the language of the neural network’s outputs. It looks for telltale signs that, with time, reveal the nature of the inputs. With each new piece of data and each response it analyzes, it better predicts the information you provide.
This process is a constant cycle of hypothesis and testing. With enough outputs, the inversion model can accurately infer a detailed profile of you, even from the most innocuous-seeming data.
The inversion model’s process is a game of connecting the dots. Each piece of data leaked through the interaction allows the model to form a profile, and with enough time, the profile it forms is unexpectedly detailed.
Eventually, insights into the user’s activities, preferences, and identity are revealed. Insights that were not meant to be disclosed or made public.
What Makes It Possible?
Within neural networks, each query and response is a data point. Skilled attackers deploy advanced statistical methods to analyze these data points and seek correlations and patterns imperceptible to human understanding.
Techniques such as regression analysis (examining the relationship between two variables) to predict the values of the input based on the outputs you receive.
Hackers use machine learning algorithms in their own inversion models to refine their predictions. They take the outputs from the chatbot and feed them into their algorithms to train them to approximate the inverse function of the target neural network.
In simplified terms, “inverse function” refers to how the hackers reverse the data flow from output to input. The goal of the attacker is to train their inversion models to perform the opposite task of the original neural network.
In essence, this is how they create a model that, given the output alone, tries to calculate what the input must have been.
How Inversion Attacks Can Be Used Against You
Imagine you’re using a popular online health assessment tool. You type in your symptoms, previous conditions, dietary habits, and even drug use to get some insight into your well-being.
That’s sensitive and personal information .
With an inversion attack targeting the AI system you’re using, a hacker might be able to take the general advice the chatbot gives you and use it to infer your private medical history. For example, a response from the chatbot might be something like this:
Antinuclear antibody (ANA) can be used to indicate the presence of autoimmune diseases such as Lupus.
The inversion model can predict that the target user was asking questions related to an autoimmune condition. With more information and more responses, the hackers can infer that the target has a serious health condition. Suddenly, the helpful online tool becomes a digital peephole into your personal health.
What Can Be Done About Inversion Attacks?
Can we build a fort around our personal data ? Well, it’s complicated. Developers of neural networks can make it tougher to carry out inversion model attacks by adding layers of security and obscuring how they operate. Here are some examples of techniques employed to protect users:
- Differential Privacy: This ensures that AI outputs are sufficiently “noisy” to mask individual data points. It’s a little like whispering in a crowd—your words are lost in the collective chatter of those around you.
- Multi-Party Computation: This technique is like a team working on a confidential project by sharing only the results of their individual tasks, not the sensitive details. It enables multiple systems to process data together without exposing individual user data to the network—or each other.
- Federated Learning: Involves training an AI across multiple devices, all while keeping individual user’s data local. It’s a little like a choir singing together; you can hear every voice, but no single voice can be isolated or identified.
While these solutions are largely effective, protecting against inversion attacks is a cat-and-mouse game. As defenses improve, so do the techniques to bypass them. The responsibility, then, falls on the companies and developers that collect and store our data, but there are ways you can protect yourself.
How to Protect Yourself Against Inversion Attacks
Image Credit:Mike MacKenzie/Flickr
Relatively speaking, neural networks and AI technologies are still in their infancy. Until the systems are foolproof, the onus is on the user to be the first line of defense when protecting your data .
Here are a few tips on how to lower the risk of becoming a victim of an inversion attack:
- Be a Selective Sharer: Treat your personal information like a secret family recipe. Be selective about who you share it with, especially when filling out forms online and interacting with chatbots. Question the necessity of every piece of data that is requested of you. If you wouldn’t share the information with a stranger, don’t share it with a chatbot.
- Keep Software Updated: Updates to front-end software, browsers, and even your operating system are designed to keep you safe . While developers are busy protecting the neural networks, you can also reduce the risk of data interception by regularly applying patches and updates.
- Keep Personal Information Personal: Whenever an application or chatbot requests personal details, pause and consider the intent. If the requested information seems irrelevant to the service provided, it probably is.
You wouldn’t provide sensitive information like health, finances, or identity to a new acquaintance just because they said they required it. Similarly, gauge what information is truly necessary for an application to function and opt out of sharing more.
Safeguarding Our Personal Information in the Age of AI
Our personal information is our most valuable asset. Guarding it requires vigilance, both in how we choose to share information and in developing security measures for the services we use.
Awareness of these threats and taking steps such as those outlined in this article contributes to a stronger defense against these seemingly invisible attack vectors.
Let’s commit to a future where our private information remains just that: private.
MUO VIDEO OF THE DAY
SCROLL TO CONTINUE WITH CONTENT
Imagine you’re at a restaurant and just tasted the best cake you’ve ever eaten. Back at your home, you’re determined to recreate this culinary masterpiece. Instead of asking for the recipe, you rely on your taste buds and knowledge to deconstruct the dessert and whip up your own.
Now, what if someone could do that with your personal information? Someone tastes the digital footprint you leave behind and reconstructs your private details.
That’s the essence of a neural network model inversion attack, a technique that could turn an AI chatbot into a cyber sleuthing tool.
Understanding Neural Network Model Inversion Attacks
A neural network is the “brain” behind modern artificial intelligence (AI). They’re responsible for the impressive functionality behind voice recognition, humanized chatbots, and generative AI.
Neural networks are essentially a series of algorithms designed to recognize patterns, think, and even learn like a human brain. They do so at a scale and speed that far surpasses our organic capabilities.
AI’s Book of Secrets
Just like our human brain, neural networks can hide secrets. These secrets are the data its users have fed them. In a model inversion attack, a hacker uses the outputs of a neural network (like the responses from a chatbot) to reverse-engineer the inputs (the information you’ve provided).
To execute the attack, hackers use their own machine learning model called an “inversion model.” This model is designed to be a mirror image of sorts, trained not on the original data but on the outputs generated by the target.
The purpose of this inversion model is to predict the inputs—the original, often sensitive data that you have fed into the chatbot.
Creating the Inversion Model
Creating the inversion can be thought of as reconstructing a shredded document. But instead of piecing together strips of paper, it’s piecing together the story told to the target model’s responses.
The inversion model learns the language of the neural network’s outputs. It looks for telltale signs that, with time, reveal the nature of the inputs. With each new piece of data and each response it analyzes, it better predicts the information you provide.
This process is a constant cycle of hypothesis and testing. With enough outputs, the inversion model can accurately infer a detailed profile of you, even from the most innocuous-seeming data.
The inversion model’s process is a game of connecting the dots. Each piece of data leaked through the interaction allows the model to form a profile, and with enough time, the profile it forms is unexpectedly detailed.
Eventually, insights into the user’s activities, preferences, and identity are revealed. Insights that were not meant to be disclosed or made public.
What Makes It Possible?
Within neural networks, each query and response is a data point. Skilled attackers deploy advanced statistical methods to analyze these data points and seek correlations and patterns imperceptible to human understanding.
Techniques such as regression analysis (examining the relationship between two variables) to predict the values of the input based on the outputs you receive.
Hackers use machine learning algorithms in their own inversion models to refine their predictions. They take the outputs from the chatbot and feed them into their algorithms to train them to approximate the inverse function of the target neural network.
In simplified terms, “inverse function” refers to how the hackers reverse the data flow from output to input. The goal of the attacker is to train their inversion models to perform the opposite task of the original neural network.
In essence, this is how they create a model that, given the output alone, tries to calculate what the input must have been.
How Inversion Attacks Can Be Used Against You
Imagine you’re using a popular online health assessment tool. You type in your symptoms, previous conditions, dietary habits, and even drug use to get some insight into your well-being.
That’s sensitive and personal information .
With an inversion attack targeting the AI system you’re using, a hacker might be able to take the general advice the chatbot gives you and use it to infer your private medical history. For example, a response from the chatbot might be something like this:
Antinuclear antibody (ANA) can be used to indicate the presence of autoimmune diseases such as Lupus.
The inversion model can predict that the target user was asking questions related to an autoimmune condition. With more information and more responses, the hackers can infer that the target has a serious health condition. Suddenly, the helpful online tool becomes a digital peephole into your personal health.
What Can Be Done About Inversion Attacks?
Can we build a fort around our personal data ? Well, it’s complicated. Developers of neural networks can make it tougher to carry out inversion model attacks by adding layers of security and obscuring how they operate. Here are some examples of techniques employed to protect users:
- Differential Privacy: This ensures that AI outputs are sufficiently “noisy” to mask individual data points. It’s a little like whispering in a crowd—your words are lost in the collective chatter of those around you.
- Multi-Party Computation: This technique is like a team working on a confidential project by sharing only the results of their individual tasks, not the sensitive details. It enables multiple systems to process data together without exposing individual user data to the network—or each other.
- Federated Learning: Involves training an AI across multiple devices, all while keeping individual user’s data local. It’s a little like a choir singing together; you can hear every voice, but no single voice can be isolated or identified.
While these solutions are largely effective, protecting against inversion attacks is a cat-and-mouse game. As defenses improve, so do the techniques to bypass them. The responsibility, then, falls on the companies and developers that collect and store our data, but there are ways you can protect yourself.
How to Protect Yourself Against Inversion Attacks
Image Credit:Mike MacKenzie/Flickr
Relatively speaking, neural networks and AI technologies are still in their infancy. Until the systems are foolproof, the onus is on the user to be the first line of defense when protecting your data .
Here are a few tips on how to lower the risk of becoming a victim of an inversion attack:
- Be a Selective Sharer: Treat your personal information like a secret family recipe. Be selective about who you share it with, especially when filling out forms online and interacting with chatbots. Question the necessity of every piece of data that is requested of you. If you wouldn’t share the information with a stranger, don’t share it with a chatbot.
- Keep Software Updated: Updates to front-end software, browsers, and even your operating system are designed to keep you safe . While developers are busy protecting the neural networks, you can also reduce the risk of data interception by regularly applying patches and updates.
- Keep Personal Information Personal: Whenever an application or chatbot requests personal details, pause and consider the intent. If the requested information seems irrelevant to the service provided, it probably is.
You wouldn’t provide sensitive information like health, finances, or identity to a new acquaintance just because they said they required it. Similarly, gauge what information is truly necessary for an application to function and opt out of sharing more.
Safeguarding Our Personal Information in the Age of AI
Our personal information is our most valuable asset. Guarding it requires vigilance, both in how we choose to share information and in developing security measures for the services we use.
Awareness of these threats and taking steps such as those outlined in this article contributes to a stronger defense against these seemingly invisible attack vectors.
Let’s commit to a future where our private information remains just that: private.
Also read:
- [New] 2024 Approved Webcam Wonders - Unveiling Tools for Top Video Quality
- [New] In 2024, Leaders in Video to File Transformation The Best iOS & PC Tools #8
- [New] YouTube Alternative Free Conversion of FB Videos to MP4 Format (HD Quality) for 2024
- [Updated] 2024 Approved Detailed Examination Intuitive HDR Techniques
- 2024 Approved Mastering Visual Storytelling with Free Images From These Esteemed Online Portals
- All About Apple Vision Pro: Unveiling Pricing & Key Features with Expert Reviews – Your Complete Guide
- How to Change Lock Screen Wallpaper on Vivo Y100 5G
- In 2024, Filmora Coupon Guide 4 Easy Ways to Redeem Exclusive Offers
- In 2024, Journey Through Ingenious Animated Texts Top 14 Snapshots
- Leading Small Tablet Devices of the Year (202#): In-Depth Expert Reviews & Ratings | ZDNET
- Memorial Day Deal Alert: Score $60 Off the Apple Watch SE - Best Buy of 2023 on ZDNet
- No Emoji Tweets, Linus's Disclosures, Trojans Explained, & ChatGPT Faults Displayed.
- Peering Into the Intelligent Machine
- Top-Rated iPad Models : In-Depth Reviews by Tech Experts - ZDNet
- Unihertz Atom XL Evaluation: Discover the Impressive Capabilities of This Compact and Durable Device
- What to Choose After iPhone 13, 15 Pro Exit the Stage - Expert Picks
- Why You Might Want to Pause on These Four Key MacBook Models - Expert Analysis by ZDNet
- Title: Preventing Oversharing: Secure Neural Networks
- Author: Brian
- Created at : 2024-11-21 16:02:59
- Updated at : 2024-11-27 16:55:53
- Link: https://tech-savvy.techidaily.com/preventing-oversharing-secure-neural-networks/
- License: This work is licensed under CC BY-NC-SA 4.0.