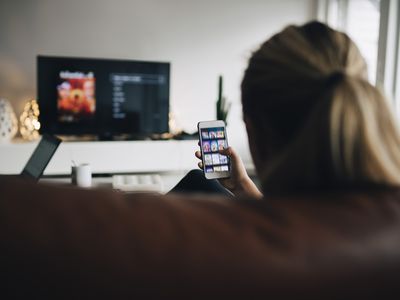
Reducing Delusionary Data in Machine Learning with Targeted Prompts

Reducing Delusionary Data in Machine Learning with Targeted Prompts
Disclaimer: This post includes affiliate links
If you click on a link and make a purchase, I may receive a commission at no extra cost to you.
Key Takeaways
- Clear and specific prompts are crucial to minimize AI hallucination. Avoid vague instructions and provide explicit details to prevent unpredictable results.
- Use grounding or the “according to…” technique to attribute output to a specific source or perspective. This helps avoid factual errors and bias in AI-generated content.
- Use constraints and rules to shape AI output according to desired outcomes. Explicitly state constraints or imply them through context or task to prevent inappropriate or illogical outputs.
MUO VIDEO OF THE DAY
SCROLL TO CONTINUE WITH CONTENT
Not getting the response you want from a generative AI model? You might be dealing with AI hallucination, a problem that occurs when the model produces inaccurate or irrelevant outputs.
It is caused by various factors, such as the quality of the data used to train the model, a lack of context, or the ambiguity of the prompt. Fortunately, there are techniques you can use to get more reliable output from an AI model.
1. Provide Clear and Specific Prompts
The first step in minimizing AI hallucination is to create clear and highly specific prompts. Vague or ambiguous prompts can lead to unpredictable results, as AI models may attempt to interpret the intent behind the prompt. Instead, be explicit in your instructions.
Instead of asking, “Tell me about dogs,” you could prompt, “Give me a detailed description of the physical characteristics and temperament of Golden Retrievers.” Refining your prompt until it’s clear is an easy way to prevent AI hallucination.
2. Use Grounding or the “According to…” Technique
One of the challenges of using AI systems is that they might generate outputs that are factually incorrect, biased, or inconsistent with your views or values. This can happen because the AI systems are trained on large and diverse datasets that might contain errors, opinions, or contradictions.
To avoid this, you can use grounding or the “according to…” technique, which involves attributing the output to a specific source or perspective. For example, you could ask the AI system to write a fact about a topic according to Wikipedia, Google Scholar, or a specific publicly accessible source.
3. Use Constraints and Rules
Constraints and rules can help prevent the AI system from generating inappropriate, inconsistent, contradictory, or illogical outputs. They can also help shape and refine the output according to the desired outcome and purpose. Constraints and rules can be explicitly stated in the prompt or implicitly implied by the context or the task.
Suppose you want to use an AI tool to write a poem about love. Instead of giving it a general prompt like “write a poem about love,” you can give it a more constrained and rule-based prompt like “write a sonnet about love with 14 lines and 10 syllables per line.”
4. Use Multi-Step Prompting
Sometimes, complex questions can lead to AI hallucinations because the model attempts to answer them in a single step. To overcome this, break down your queries into multiple steps.
For instance, instead of asking, “What is the most effective diabetes treatment?” you can ask, “What are the common treatments for diabetes?” You can then follow up with, “Which of these treatments is considered the most effective according to medical studies?”
Multi-step prompting forces the AI model to provide intermediate information before arriving at a final answer, which can lead to more accurate and well-informed responses.
5. Assign Role to AI
When you assign a specific role to the AI model in your prompt, you clarify its purpose and reduce the likelihood of hallucination. For example, instead of saying, “Tell me about the history of quantum mechanics,” you can prompt the AI with, “Assume the role of a diligent researcher and provide a summary of the key milestones in the history of quantum mechanics.”
This framing encourages the AI to act as a diligent researcher rather than a speculative storyteller.
6. Add Contextual Information
Not providing contextual information when necessary is a prompt mistake to avoid when using ChatGPT or other AI models. Contextual information helps the model understand the task’s background, domain, or purpose and generate more relevant and coherent outputs. Contextual information includes keywords, tags, categories, examples, references, and sources.
For example, if you want to generate a product review for a pair of headphones, you can provide contextual information, such as the product name, brand, features, price, rating, or customer feedback. A good prompt for this task could look something like this:
Getting Better AI Responses
It can be frustrating when you don’t get the feedback you expect from an AI model. However, using these AI prompting techniques, you can reduce the likelihood of AI hallucination and get better and more reliable responses from your AI systems.
Keep in mind that these techniques are not foolproof and may not work for every task or topic. You should always check and verify the AI outputs before using them for any serious purpose.
MUO VIDEO OF THE DAY
SCROLL TO CONTINUE WITH CONTENT
Not getting the response you want from a generative AI model? You might be dealing with AI hallucination, a problem that occurs when the model produces inaccurate or irrelevant outputs.
It is caused by various factors, such as the quality of the data used to train the model, a lack of context, or the ambiguity of the prompt. Fortunately, there are techniques you can use to get more reliable output from an AI model.
1. Provide Clear and Specific Prompts
The first step in minimizing AI hallucination is to create clear and highly specific prompts. Vague or ambiguous prompts can lead to unpredictable results, as AI models may attempt to interpret the intent behind the prompt. Instead, be explicit in your instructions.
Instead of asking, “Tell me about dogs,” you could prompt, “Give me a detailed description of the physical characteristics and temperament of Golden Retrievers.” Refining your prompt until it’s clear is an easy way to prevent AI hallucination.
2. Use Grounding or the “According to…” Technique
One of the challenges of using AI systems is that they might generate outputs that are factually incorrect, biased, or inconsistent with your views or values. This can happen because the AI systems are trained on large and diverse datasets that might contain errors, opinions, or contradictions.
To avoid this, you can use grounding or the “according to…” technique, which involves attributing the output to a specific source or perspective. For example, you could ask the AI system to write a fact about a topic according to Wikipedia, Google Scholar, or a specific publicly accessible source.
3. Use Constraints and Rules
Constraints and rules can help prevent the AI system from generating inappropriate, inconsistent, contradictory, or illogical outputs. They can also help shape and refine the output according to the desired outcome and purpose. Constraints and rules can be explicitly stated in the prompt or implicitly implied by the context or the task.
Suppose you want to use an AI tool to write a poem about love. Instead of giving it a general prompt like “write a poem about love,” you can give it a more constrained and rule-based prompt like “write a sonnet about love with 14 lines and 10 syllables per line.”
4. Use Multi-Step Prompting
Sometimes, complex questions can lead to AI hallucinations because the model attempts to answer them in a single step. To overcome this, break down your queries into multiple steps.
For instance, instead of asking, “What is the most effective diabetes treatment?” you can ask, “What are the common treatments for diabetes?” You can then follow up with, “Which of these treatments is considered the most effective according to medical studies?”
Multi-step prompting forces the AI model to provide intermediate information before arriving at a final answer, which can lead to more accurate and well-informed responses.
5. Assign Role to AI
When you assign a specific role to the AI model in your prompt, you clarify its purpose and reduce the likelihood of hallucination. For example, instead of saying, “Tell me about the history of quantum mechanics,” you can prompt the AI with, “Assume the role of a diligent researcher and provide a summary of the key milestones in the history of quantum mechanics.”
This framing encourages the AI to act as a diligent researcher rather than a speculative storyteller.
6. Add Contextual Information
Not providing contextual information when necessary is a prompt mistake to avoid when using ChatGPT or other AI models. Contextual information helps the model understand the task’s background, domain, or purpose and generate more relevant and coherent outputs. Contextual information includes keywords, tags, categories, examples, references, and sources.
For example, if you want to generate a product review for a pair of headphones, you can provide contextual information, such as the product name, brand, features, price, rating, or customer feedback. A good prompt for this task could look something like this:
Getting Better AI Responses
It can be frustrating when you don’t get the feedback you expect from an AI model. However, using these AI prompting techniques, you can reduce the likelihood of AI hallucination and get better and more reliable responses from your AI systems.
Keep in mind that these techniques are not foolproof and may not work for every task or topic. You should always check and verify the AI outputs before using them for any serious purpose.
MUO VIDEO OF THE DAY
SCROLL TO CONTINUE WITH CONTENT
Not getting the response you want from a generative AI model? You might be dealing with AI hallucination, a problem that occurs when the model produces inaccurate or irrelevant outputs.
It is caused by various factors, such as the quality of the data used to train the model, a lack of context, or the ambiguity of the prompt. Fortunately, there are techniques you can use to get more reliable output from an AI model.
1. Provide Clear and Specific Prompts
The first step in minimizing AI hallucination is to create clear and highly specific prompts. Vague or ambiguous prompts can lead to unpredictable results, as AI models may attempt to interpret the intent behind the prompt. Instead, be explicit in your instructions.
Instead of asking, “Tell me about dogs,” you could prompt, “Give me a detailed description of the physical characteristics and temperament of Golden Retrievers.” Refining your prompt until it’s clear is an easy way to prevent AI hallucination.
2. Use Grounding or the “According to…” Technique
One of the challenges of using AI systems is that they might generate outputs that are factually incorrect, biased, or inconsistent with your views or values. This can happen because the AI systems are trained on large and diverse datasets that might contain errors, opinions, or contradictions.
To avoid this, you can use grounding or the “according to…” technique, which involves attributing the output to a specific source or perspective. For example, you could ask the AI system to write a fact about a topic according to Wikipedia, Google Scholar, or a specific publicly accessible source.
3. Use Constraints and Rules
Constraints and rules can help prevent the AI system from generating inappropriate, inconsistent, contradictory, or illogical outputs. They can also help shape and refine the output according to the desired outcome and purpose. Constraints and rules can be explicitly stated in the prompt or implicitly implied by the context or the task.
Suppose you want to use an AI tool to write a poem about love. Instead of giving it a general prompt like “write a poem about love,” you can give it a more constrained and rule-based prompt like “write a sonnet about love with 14 lines and 10 syllables per line.”
4. Use Multi-Step Prompting
Sometimes, complex questions can lead to AI hallucinations because the model attempts to answer them in a single step. To overcome this, break down your queries into multiple steps.
For instance, instead of asking, “What is the most effective diabetes treatment?” you can ask, “What are the common treatments for diabetes?” You can then follow up with, “Which of these treatments is considered the most effective according to medical studies?”
Multi-step prompting forces the AI model to provide intermediate information before arriving at a final answer, which can lead to more accurate and well-informed responses.
5. Assign Role to AI
When you assign a specific role to the AI model in your prompt, you clarify its purpose and reduce the likelihood of hallucination. For example, instead of saying, “Tell me about the history of quantum mechanics,” you can prompt the AI with, “Assume the role of a diligent researcher and provide a summary of the key milestones in the history of quantum mechanics.”
This framing encourages the AI to act as a diligent researcher rather than a speculative storyteller.
6. Add Contextual Information
Not providing contextual information when necessary is a prompt mistake to avoid when using ChatGPT or other AI models. Contextual information helps the model understand the task’s background, domain, or purpose and generate more relevant and coherent outputs. Contextual information includes keywords, tags, categories, examples, references, and sources.
For example, if you want to generate a product review for a pair of headphones, you can provide contextual information, such as the product name, brand, features, price, rating, or customer feedback. A good prompt for this task could look something like this:
Getting Better AI Responses
It can be frustrating when you don’t get the feedback you expect from an AI model. However, using these AI prompting techniques, you can reduce the likelihood of AI hallucination and get better and more reliable responses from your AI systems.
Keep in mind that these techniques are not foolproof and may not work for every task or topic. You should always check and verify the AI outputs before using them for any serious purpose.
MUO VIDEO OF THE DAY
SCROLL TO CONTINUE WITH CONTENT
Not getting the response you want from a generative AI model? You might be dealing with AI hallucination, a problem that occurs when the model produces inaccurate or irrelevant outputs.
It is caused by various factors, such as the quality of the data used to train the model, a lack of context, or the ambiguity of the prompt. Fortunately, there are techniques you can use to get more reliable output from an AI model.
1. Provide Clear and Specific Prompts
The first step in minimizing AI hallucination is to create clear and highly specific prompts. Vague or ambiguous prompts can lead to unpredictable results, as AI models may attempt to interpret the intent behind the prompt. Instead, be explicit in your instructions.
Instead of asking, “Tell me about dogs,” you could prompt, “Give me a detailed description of the physical characteristics and temperament of Golden Retrievers.” Refining your prompt until it’s clear is an easy way to prevent AI hallucination.
2. Use Grounding or the “According to…” Technique
One of the challenges of using AI systems is that they might generate outputs that are factually incorrect, biased, or inconsistent with your views or values. This can happen because the AI systems are trained on large and diverse datasets that might contain errors, opinions, or contradictions.
To avoid this, you can use grounding or the “according to…” technique, which involves attributing the output to a specific source or perspective. For example, you could ask the AI system to write a fact about a topic according to Wikipedia, Google Scholar, or a specific publicly accessible source.
3. Use Constraints and Rules
Constraints and rules can help prevent the AI system from generating inappropriate, inconsistent, contradictory, or illogical outputs. They can also help shape and refine the output according to the desired outcome and purpose. Constraints and rules can be explicitly stated in the prompt or implicitly implied by the context or the task.
Suppose you want to use an AI tool to write a poem about love. Instead of giving it a general prompt like “write a poem about love,” you can give it a more constrained and rule-based prompt like “write a sonnet about love with 14 lines and 10 syllables per line.”
4. Use Multi-Step Prompting
Sometimes, complex questions can lead to AI hallucinations because the model attempts to answer them in a single step. To overcome this, break down your queries into multiple steps.
For instance, instead of asking, “What is the most effective diabetes treatment?” you can ask, “What are the common treatments for diabetes?” You can then follow up with, “Which of these treatments is considered the most effective according to medical studies?”
Multi-step prompting forces the AI model to provide intermediate information before arriving at a final answer, which can lead to more accurate and well-informed responses.
5. Assign Role to AI
When you assign a specific role to the AI model in your prompt, you clarify its purpose and reduce the likelihood of hallucination. For example, instead of saying, “Tell me about the history of quantum mechanics,” you can prompt the AI with, “Assume the role of a diligent researcher and provide a summary of the key milestones in the history of quantum mechanics.”
This framing encourages the AI to act as a diligent researcher rather than a speculative storyteller.
6. Add Contextual Information
Not providing contextual information when necessary is a prompt mistake to avoid when using ChatGPT or other AI models. Contextual information helps the model understand the task’s background, domain, or purpose and generate more relevant and coherent outputs. Contextual information includes keywords, tags, categories, examples, references, and sources.
For example, if you want to generate a product review for a pair of headphones, you can provide contextual information, such as the product name, brand, features, price, rating, or customer feedback. A good prompt for this task could look something like this:
Getting Better AI Responses
It can be frustrating when you don’t get the feedback you expect from an AI model. However, using these AI prompting techniques, you can reduce the likelihood of AI hallucination and get better and more reliable responses from your AI systems.
Keep in mind that these techniques are not foolproof and may not work for every task or topic. You should always check and verify the AI outputs before using them for any serious purpose.
Also read:
- [Updated] 2024 Approved Key Aspects of ZDSoft Vision Recorder
- [Updated] In 2024, Dominate the Digital Arena Audience Expansion on Youtube
- [Updated] IPhone Mastery Complete Guide to Capturing Podcasts for 2024
- [Updated] Step Into a TikTok Realm A Guide for Joining Lives
- Beyond Expectations: How the Ring Spotlight Cam Pro Blew My Mind | Tech Insights From ZDNET
- Black Friday Deal: Jackery Explorer 1000 Generator Discounted by $250 - Save Now!
- Capturing the Spectacular 2024 Total Solar Eclipse: A Journey From Onset to Culmination with Exclusive Images - ZealTech
- Celebrate the Ultimate Grass-Trimming Joy with Our Remote-Controlled Lawnmower | ZDNet
- Crafting a Blueprint for Locating Captivating Pexel Visuals for 2024
- Discount Alert: Enhance Your Home with GE's Cync Collection - Lights and Switches at Up to 61% Off | ZDNET
- How to Unlock Itel A60 PIN Code/Pattern Lock/Password
- In 2024, How To Remove Screen Lock PIN On Infinix Zero 5G 2023 Turbo Like A Pro 5 Easy Ways
- Perfect iPhone Silhouette Captures 101 for 2024
- Resolve PC Lag in Resident Evil Village with These Proven Fixes - Guide Released!
- Title: Reducing Delusionary Data in Machine Learning with Targeted Prompts
- Author: Brian
- Created at : 2024-12-22 16:51:32
- Updated at : 2024-12-27 16:39:57
- Link: https://tech-savvy.techidaily.com/reducing-delusionary-data-in-machine-learning-with-targeted-prompts/
- License: This work is licensed under CC BY-NC-SA 4.0.