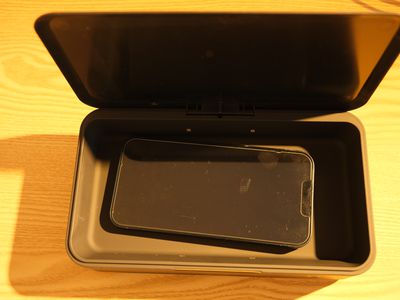
The Evolutionary Path: Advanced AI Conversations

The Evolutionary Path: Advanced AI Conversations
Disclaimer: This post includes affiliate links
If you click on a link and make a purchase, I may receive a commission at no extra cost to you.
Key Takeaways
- ChatGPT’s success has triggered widespread investment in AI research and integration, leading to unprecedented opportunities and advancements in the field.
- Semantic search with vector databases is revolutionizing search algorithms by utilizing word embeddings and semantics to provide more contextually accurate results.
- The development of AI agents and multi-agent startups aims to achieve full autonomy and resolve current limitations through self-assessment, correction, and collaboration among multiple agents.
MUO VIDEO OF THE DAY
SCROLL TO CONTINUE WITH CONTENT
ChatGPT’s phenomenal success has forced every tech company to start investing in AI research and figure out how to integrate artificial intelligence into their products. It’s a situation unlike anything we’ve ever seen, yet, artificial intelligence is only just getting started.
But it’s not just about fancy AI chatbots and text-to-image generators. There are some highly speculation but incredibly impressive AI tools on the horizon.
Semantic Search With Vector Databases
Image Credit:Firmbee.com/Unsplash
Semantic search queries are being tested to provide better search results for people. Search engines currently use keyword-centric algorithms to provide relevant information to users. However, overreliance on keywords poses several problems, such as limited context understanding, marketers exploiting SEO, and low-quality search results due to difficulty expressing complex queries.
Unlike traditional search algorithms, semantic search uses word embeddings and semantic mapping to understand the context of a query before providing search results. So, instead of relying on a bunch of keywords, semantic search provides results based on semantics or the meaning of a given query.
The concept of semantic search has been around for quite some time. However, companies have difficulty implementing such functionality due to how slow and resource-intensive semantic search can be.
The solution is to map out vector embeddings and store them in a large vector database . Doing so substantially lowers computing power requirements and speeds up search results by narrowing results to only the most relevant information.
Large tech companies and startups like Pinecone, Redis, and Milvus are currently investing in vector databases to provide semantic search capabilities on recommendation systems, search engines, content management systems, and chatbots.
Democratization of AI
Although not necessarily a technical advancement, several big tech companies are interested in democratizing AI. For better or for worse, open-source AI models are now being trained and given more permissive licenses for organizations to use and fine-tune.
The Wall Street Journal reports that Meta is buying Nvidia H100 AI accelerators and aims to develop an AI that competes with OpenAI’s recent GPT-4 model.
There is currently no publicly available LLM that can match the raw performance of GPT-4. But with Meta promising a competitive product with a more permissive license, companies can finally fine-tune a powerful LLM without the risk of trade secrets and sensitive data being exposed and used against them.
AI Agents and Multi-Agent Startups
Image Credit:Annie Spratt/Unsplash
Several experimental projects are currently in the works for developing AI agents that require little to no instructions to achieve a certain goal. You may remember the concepts of AI agents from Auto-GPT , the AI tool that automates its actions.
The idea is for the agent to attain full autonomy through constant self-assessment and self-correction. The working concept to achieve self-reflection and correction is for the agent to continually prompt itself every step of the way on what action needs to be done, steps on how to do it, what mistakes it made, and what it can do to improve.
The problem is that the current models used in AI agents have little semantic understanding. That causes the agents to hallucinate and prompt false information, which causes them to get stuck on an infinite loop of self-assessment and correction.
Projects like the MetaGPT Multi-agent Framework aim to solve the problem by simultaneously using several AI agents to reduce such hallucinations. Multi-agent frameworks are set up to emulate how a startup company would work. Each agent in this startup will be assigned positions such as project manager, project designer, programmer, and tester. By splitting complex goals into smaller tasks and delegating them to different AI agents, these agents are more likely to achieve their given goals.
Of course, these frameworks are still very early in development, and many issues still need to be solved. But with more powerful models, better AI infrastructure, and continuous research and development, it is only a matter of time before effective AI agents and multi-agent AI companies become a thing.
Shaping Our Future With AI
Large corporations and startups are heavily investing in the research and development of AI and its infrastructures. So, we can expect the future of generative AI to provide better access to useful information through semantic search, fully autonomous AI agents and AI companies, and freely available high-performance models for companies and individuals to use and fine-tune.
Although exciting, it is also important that we take our time to consider AI ethics, user privacy, and the responsible development of AI systems and infrastructures. Let us remember that the evolution of generative AI is not just about building smarter systems; it is also about reshaping our thoughts and being responsible for the way we use technology.
MUO VIDEO OF THE DAY
SCROLL TO CONTINUE WITH CONTENT
ChatGPT’s phenomenal success has forced every tech company to start investing in AI research and figure out how to integrate artificial intelligence into their products. It’s a situation unlike anything we’ve ever seen, yet, artificial intelligence is only just getting started.
But it’s not just about fancy AI chatbots and text-to-image generators. There are some highly speculation but incredibly impressive AI tools on the horizon.
Semantic Search With Vector Databases
Image Credit:Firmbee.com/Unsplash
Semantic search queries are being tested to provide better search results for people. Search engines currently use keyword-centric algorithms to provide relevant information to users. However, overreliance on keywords poses several problems, such as limited context understanding, marketers exploiting SEO, and low-quality search results due to difficulty expressing complex queries.
Unlike traditional search algorithms, semantic search uses word embeddings and semantic mapping to understand the context of a query before providing search results. So, instead of relying on a bunch of keywords, semantic search provides results based on semantics or the meaning of a given query.
The concept of semantic search has been around for quite some time. However, companies have difficulty implementing such functionality due to how slow and resource-intensive semantic search can be.
The solution is to map out vector embeddings and store them in a large vector database . Doing so substantially lowers computing power requirements and speeds up search results by narrowing results to only the most relevant information.
Large tech companies and startups like Pinecone, Redis, and Milvus are currently investing in vector databases to provide semantic search capabilities on recommendation systems, search engines, content management systems, and chatbots.
Democratization of AI
Although not necessarily a technical advancement, several big tech companies are interested in democratizing AI. For better or for worse, open-source AI models are now being trained and given more permissive licenses for organizations to use and fine-tune.
The Wall Street Journal reports that Meta is buying Nvidia H100 AI accelerators and aims to develop an AI that competes with OpenAI’s recent GPT-4 model.
There is currently no publicly available LLM that can match the raw performance of GPT-4. But with Meta promising a competitive product with a more permissive license, companies can finally fine-tune a powerful LLM without the risk of trade secrets and sensitive data being exposed and used against them.
AI Agents and Multi-Agent Startups
Image Credit:Annie Spratt/Unsplash
Several experimental projects are currently in the works for developing AI agents that require little to no instructions to achieve a certain goal. You may remember the concepts of AI agents from Auto-GPT , the AI tool that automates its actions.
The idea is for the agent to attain full autonomy through constant self-assessment and self-correction. The working concept to achieve self-reflection and correction is for the agent to continually prompt itself every step of the way on what action needs to be done, steps on how to do it, what mistakes it made, and what it can do to improve.
The problem is that the current models used in AI agents have little semantic understanding. That causes the agents to hallucinate and prompt false information, which causes them to get stuck on an infinite loop of self-assessment and correction.
Projects like the MetaGPT Multi-agent Framework aim to solve the problem by simultaneously using several AI agents to reduce such hallucinations. Multi-agent frameworks are set up to emulate how a startup company would work. Each agent in this startup will be assigned positions such as project manager, project designer, programmer, and tester. By splitting complex goals into smaller tasks and delegating them to different AI agents, these agents are more likely to achieve their given goals.
Of course, these frameworks are still very early in development, and many issues still need to be solved. But with more powerful models, better AI infrastructure, and continuous research and development, it is only a matter of time before effective AI agents and multi-agent AI companies become a thing.
Shaping Our Future With AI
Large corporations and startups are heavily investing in the research and development of AI and its infrastructures. So, we can expect the future of generative AI to provide better access to useful information through semantic search, fully autonomous AI agents and AI companies, and freely available high-performance models for companies and individuals to use and fine-tune.
Although exciting, it is also important that we take our time to consider AI ethics, user privacy, and the responsible development of AI systems and infrastructures. Let us remember that the evolution of generative AI is not just about building smarter systems; it is also about reshaping our thoughts and being responsible for the way we use technology.
MUO VIDEO OF THE DAY
SCROLL TO CONTINUE WITH CONTENT
ChatGPT’s phenomenal success has forced every tech company to start investing in AI research and figure out how to integrate artificial intelligence into their products. It’s a situation unlike anything we’ve ever seen, yet, artificial intelligence is only just getting started.
But it’s not just about fancy AI chatbots and text-to-image generators. There are some highly speculation but incredibly impressive AI tools on the horizon.
Semantic Search With Vector Databases
Image Credit:Firmbee.com/Unsplash
Semantic search queries are being tested to provide better search results for people. Search engines currently use keyword-centric algorithms to provide relevant information to users. However, overreliance on keywords poses several problems, such as limited context understanding, marketers exploiting SEO, and low-quality search results due to difficulty expressing complex queries.
Unlike traditional search algorithms, semantic search uses word embeddings and semantic mapping to understand the context of a query before providing search results. So, instead of relying on a bunch of keywords, semantic search provides results based on semantics or the meaning of a given query.
The concept of semantic search has been around for quite some time. However, companies have difficulty implementing such functionality due to how slow and resource-intensive semantic search can be.
The solution is to map out vector embeddings and store them in a large vector database . Doing so substantially lowers computing power requirements and speeds up search results by narrowing results to only the most relevant information.
Large tech companies and startups like Pinecone, Redis, and Milvus are currently investing in vector databases to provide semantic search capabilities on recommendation systems, search engines, content management systems, and chatbots.
Democratization of AI
Although not necessarily a technical advancement, several big tech companies are interested in democratizing AI. For better or for worse, open-source AI models are now being trained and given more permissive licenses for organizations to use and fine-tune.
The Wall Street Journal reports that Meta is buying Nvidia H100 AI accelerators and aims to develop an AI that competes with OpenAI’s recent GPT-4 model.
There is currently no publicly available LLM that can match the raw performance of GPT-4. But with Meta promising a competitive product with a more permissive license, companies can finally fine-tune a powerful LLM without the risk of trade secrets and sensitive data being exposed and used against them.
AI Agents and Multi-Agent Startups
Image Credit:Annie Spratt/Unsplash
Several experimental projects are currently in the works for developing AI agents that require little to no instructions to achieve a certain goal. You may remember the concepts of AI agents from Auto-GPT , the AI tool that automates its actions.
The idea is for the agent to attain full autonomy through constant self-assessment and self-correction. The working concept to achieve self-reflection and correction is for the agent to continually prompt itself every step of the way on what action needs to be done, steps on how to do it, what mistakes it made, and what it can do to improve.
The problem is that the current models used in AI agents have little semantic understanding. That causes the agents to hallucinate and prompt false information, which causes them to get stuck on an infinite loop of self-assessment and correction.
Projects like the MetaGPT Multi-agent Framework aim to solve the problem by simultaneously using several AI agents to reduce such hallucinations. Multi-agent frameworks are set up to emulate how a startup company would work. Each agent in this startup will be assigned positions such as project manager, project designer, programmer, and tester. By splitting complex goals into smaller tasks and delegating them to different AI agents, these agents are more likely to achieve their given goals.
Of course, these frameworks are still very early in development, and many issues still need to be solved. But with more powerful models, better AI infrastructure, and continuous research and development, it is only a matter of time before effective AI agents and multi-agent AI companies become a thing.
Shaping Our Future With AI
Large corporations and startups are heavily investing in the research and development of AI and its infrastructures. So, we can expect the future of generative AI to provide better access to useful information through semantic search, fully autonomous AI agents and AI companies, and freely available high-performance models for companies and individuals to use and fine-tune.
Although exciting, it is also important that we take our time to consider AI ethics, user privacy, and the responsible development of AI systems and infrastructures. Let us remember that the evolution of generative AI is not just about building smarter systems; it is also about reshaping our thoughts and being responsible for the way we use technology.
MUO VIDEO OF THE DAY
SCROLL TO CONTINUE WITH CONTENT
ChatGPT’s phenomenal success has forced every tech company to start investing in AI research and figure out how to integrate artificial intelligence into their products. It’s a situation unlike anything we’ve ever seen, yet, artificial intelligence is only just getting started.
But it’s not just about fancy AI chatbots and text-to-image generators. There are some highly speculation but incredibly impressive AI tools on the horizon.
Semantic Search With Vector Databases
Image Credit:Firmbee.com/Unsplash
Semantic search queries are being tested to provide better search results for people. Search engines currently use keyword-centric algorithms to provide relevant information to users. However, overreliance on keywords poses several problems, such as limited context understanding, marketers exploiting SEO, and low-quality search results due to difficulty expressing complex queries.
Unlike traditional search algorithms, semantic search uses word embeddings and semantic mapping to understand the context of a query before providing search results. So, instead of relying on a bunch of keywords, semantic search provides results based on semantics or the meaning of a given query.
The concept of semantic search has been around for quite some time. However, companies have difficulty implementing such functionality due to how slow and resource-intensive semantic search can be.
The solution is to map out vector embeddings and store them in a large vector database . Doing so substantially lowers computing power requirements and speeds up search results by narrowing results to only the most relevant information.
Large tech companies and startups like Pinecone, Redis, and Milvus are currently investing in vector databases to provide semantic search capabilities on recommendation systems, search engines, content management systems, and chatbots.
Democratization of AI
Although not necessarily a technical advancement, several big tech companies are interested in democratizing AI. For better or for worse, open-source AI models are now being trained and given more permissive licenses for organizations to use and fine-tune.
The Wall Street Journal reports that Meta is buying Nvidia H100 AI accelerators and aims to develop an AI that competes with OpenAI’s recent GPT-4 model.
There is currently no publicly available LLM that can match the raw performance of GPT-4. But with Meta promising a competitive product with a more permissive license, companies can finally fine-tune a powerful LLM without the risk of trade secrets and sensitive data being exposed and used against them.
AI Agents and Multi-Agent Startups
Image Credit:Annie Spratt/Unsplash
Several experimental projects are currently in the works for developing AI agents that require little to no instructions to achieve a certain goal. You may remember the concepts of AI agents from Auto-GPT , the AI tool that automates its actions.
The idea is for the agent to attain full autonomy through constant self-assessment and self-correction. The working concept to achieve self-reflection and correction is for the agent to continually prompt itself every step of the way on what action needs to be done, steps on how to do it, what mistakes it made, and what it can do to improve.
The problem is that the current models used in AI agents have little semantic understanding. That causes the agents to hallucinate and prompt false information, which causes them to get stuck on an infinite loop of self-assessment and correction.
Projects like the MetaGPT Multi-agent Framework aim to solve the problem by simultaneously using several AI agents to reduce such hallucinations. Multi-agent frameworks are set up to emulate how a startup company would work. Each agent in this startup will be assigned positions such as project manager, project designer, programmer, and tester. By splitting complex goals into smaller tasks and delegating them to different AI agents, these agents are more likely to achieve their given goals.
Of course, these frameworks are still very early in development, and many issues still need to be solved. But with more powerful models, better AI infrastructure, and continuous research and development, it is only a matter of time before effective AI agents and multi-agent AI companies become a thing.
Shaping Our Future With AI
Large corporations and startups are heavily investing in the research and development of AI and its infrastructures. So, we can expect the future of generative AI to provide better access to useful information through semantic search, fully autonomous AI agents and AI companies, and freely available high-performance models for companies and individuals to use and fine-tune.
Although exciting, it is also important that we take our time to consider AI ethics, user privacy, and the responsible development of AI systems and infrastructures. Let us remember that the evolution of generative AI is not just about building smarter systems; it is also about reshaping our thoughts and being responsible for the way we use technology.
Also read:
- [New] 2024 Approved Foremost 4K Camcorders Our Best Picks
- [New] In 2024, Making the Invisible Audible Fixes for Tweeted Videos
- [New] In 2024, Ultimate Laptop ScreenCapture Techniques Reviewed
- [New] In 2024, Unveiling Nature's Digital Canvas A Novice's Starter on Green Screen Technology
- [New] Peek Into Innovation with Microsoft's HoloLens Review
- [Updated] In 2024, Essential Guide to Aspect Ratios for Effective YouTube Engagement
- [Updated] In 2024, Prime 6 Bargains for Your Home's Ultimate Viewing
- AI-Infused Inspiration: 5 Groundbreaking Writing Tools
- Anticipating the Arrival: My Insightful Encounter with Early Orders for Apple Vision Pro - Indications of Improved Future Releases
- Discover the Upgraded Apple CarPlay Features Showcased at WWDC 2024: In-Depth Analysis and Expert Insights | ZDNet
- How to Assess if Your iPhone Fits in Upgrade Echelons
- How to Change Your Realme 11X 5G Location on life360 Without Anyone Knowing? | Dr.fone
- IOS 18 Release: Complete Guide on Downloading and Compatible iPhone Models - Detailed Steps From ZDNet
- IPhone Showdown: Comparing iPhone 15 Pro and iPhone 13 Pro – Making an Informed Upgrade Decision | Digital Gadget Review
- Top-Rated AirTag Wallets in 2^ - Our Experts' Comprehensive Guide & Reviews
- Title: The Evolutionary Path: Advanced AI Conversations
- Author: Brian
- Created at : 2024-10-31 11:19:16
- Updated at : 2024-11-07 12:11:36
- Link: https://tech-savvy.techidaily.com/the-evolutionary-path-advanced-ai-conversations/
- License: This work is licensed under CC BY-NC-SA 4.0.