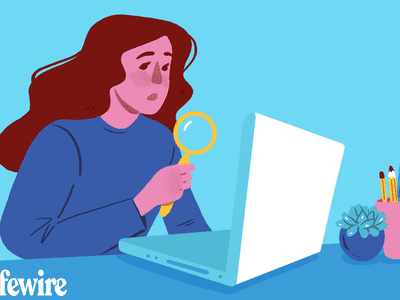
The Language Titans: Dissecting BERT and GPT's Roles

The Language Titans: Dissecting BERT and GPT’s Roles
AI tools like ChatGPT have become incredibly popular since they were released. Such tools push the boundaries of natural language processing (NLP), making it easier for AI to hold conversations and process language just like an actual person.
MUO VIDEO OF THE DAY
SCROLL TO CONTINUE WITH CONTENT
As you may know, ChatGPT relies on the Generative Pre-trained Transformer model (GPT). However, that’s not the only pre-trained model out there.
In 2018, the engineers at Google developed BERT (Bidirectional Encoder Representation from Transformers), a pre-trained, deep learning model designed to understand the context of words in a sentence, allowing it to perform tasks such as sentiment analysis, question-answering, and named entity recognition with high accuracy.
Disclaimer: This post includes affiliate links
If you click on a link and make a purchase, I may receive a commission at no extra cost to you.
What Is BERT?
BERT is a deep learning model developed by Google AI Research that uses unsupervised learning to understand natural language queries better. The model uses a transformer architecture to learn bidirectional representations of text data, which allows it to better understand the context of words within a sentence or paragraph.
This makes it easier for machines to interpret human language as spoken in everyday life. It’s important to mention that computers have historically found it difficult to process language, especially understanding the context.
Unlike other language processing models, BERT is trained to perform more than 11 common NLP tasks, making it an extremely popular choice in machine learning circles.
When compared with other popular transformer models like GPT-3, BERT has a distinct advantage: it is bidirectional and, as such, is capable of evaluating context from left to right and right to left. GPT-3.5 and GPT-4 only consider the left to right context, while BERT caters to both.
Language models like GPT use unidirectional context to train the model, allowing ChatGPT to perform several tasks. In simple terms, these models analyzed the context of text input from left to right or, in some cases, right to left. However, this unidirectional approach has limitations when it comes to text understanding, causing inaccuracies in generated outputs.
Essentially, this means that BERT analyzes a sentence’s full context before providing an answer. However, it’s pertinent to mention that GPT-3 was trained on a considerably larger corpus of text (45TB) compared to BERT (3TB).
BERT Is a Masked Language Model
An important thing to know here is that BERT relies on masking to understand the context of a sentence. When processing a sentence, it removes parts of it and relies on the model to predict and complete the gaps.
This allows it to “predict” the context, essentially. In sentences where one word can have two different meanings, this gives masked language models a distinct advantage.
How Does BERT Work?
BERT was trained on a dataset of over 3.3 billion words (relying on Wikipedia for up to 2.5 billion words) and the BooksCorpus from Google for 800 million words.
BERT’s unique bidirectional context enables the simultaneous processing of text from left to right and vice versa. This innovation enhances the model’s understanding of human language, allowing it to comprehend complex relationships between words and their context.
The bidirectionality element has positioned BERT as a revolutionary transformer model, driving remarkable improvements in NLP tasks. More importantly, it also helps outline the sheer prowess of tools that use artificial intelligence (AI) to process language.
BERT’s effectiveness is not only because of its bidirectionality but also because of how it was pre-trained. BERT’s pre-training phase comprised two essential steps, namely masked language model (MLM) and next sentence prediction (NSP).
While most pre-training methods mask individual sequence elements, BERT uses MLM to randomly mask a percentage of input tokens in a sentence during training. This approach forces the model to predict the missing words, taking into account the context from both sides of the masked word—hence the bidirectionality.
Then, during NSP, BERT learns to predict whether sentence X genuinely follows into sentence Y. This capability trains the model to understand sentence relationships and overall context, which, in turn, contributes to the model’s effectiveness.
Fine-Tuning BERT
After pre-training, BERT moved on to a fine-tuning phase, where the model was adapted to various NLP tasks, including sentiment analysis, named entity recognition, and question-answering systems. Fine-tuning involves supervised learning, leveraging labeled data sets to enhance model performance for specific tasks.
BERT’s training approach is considered “universal” because it allows the same model architecture to tackle different tasks without the need for extensive modifications. This versatility is yet another reason for BERT’s popularity among NLP enthusiasts.
For instance, BERT is used by Google to predict search queries and to plug in missing words, especially in terms of context.
What Is BERT Commonly Used For?
While Google uses BERT in its search engine, it has several other applications:
Sentiment Analysis
Sentiment analysis is a core application of NLP that deals with classifying text data based on the emotions and opinions embedded in them. This is crucial in numerous fields, from monitoring customer satisfaction to predicting stock market trends.
BERT shines in this domain, as it captures the emotional essence of textual input and accurately predicts the sentiment behind the words.
Text Summarization
Due to its bidirectional nature and attention mechanisms, BERT can grasp every iota of textual context without losing essential information. The result is high-quality, coherent summaries that accurately reflect the significant content of the input documents.
Named Entity Recognition
Named entity recognition (NER) is another vital aspect of NLP aimed at identifying and categorizing entities like names, organizations, and locations within text data.
BERT is truly transformative in the NER space, primarily because of its ability to recognize and classify complex entity patterns—even when presented within intricate text structures.
Question-Answering Systems
BERT’s contextual understanding and grounding in bidirectional encoders make it adept at extracting accurate answers from large data sets.
It can effectively determine the context of a question and locate the most suitable answer within the text data, a capability that can be harnessed for advanced chatbots, search engines, and even virtual assistants.
Machine Translation via BERT
Machine translation is an essential NLP task that BERT has improved. The transformer architecture and the bidirectional understanding of context contribute to breaking the barriers in translating from one language to another.
While primarily focused on English, BERT’s multilingual variants (mBERT) can be applied to machine translation problems for numerous languages, opening up doors to more inclusive platforms and communication mediums.
AI and Machine Learning Continue to Push New Boundaries
There’s little doubt that models such as BERT are changing the game and opening new avenues of research. But, more importantly, such tools can be easily integrated into existing workflows.
SCROLL TO CONTINUE WITH CONTENT
As you may know, ChatGPT relies on the Generative Pre-trained Transformer model (GPT). However, that’s not the only pre-trained model out there.
In 2018, the engineers at Google developed BERT (Bidirectional Encoder Representation from Transformers), a pre-trained, deep learning model designed to understand the context of words in a sentence, allowing it to perform tasks such as sentiment analysis, question-answering, and named entity recognition with high accuracy.
Also read:
- [New] Master Your Memories Online Diverse, Cost-Effective Cloud Storage Choices
- [Updated] 2024 Approved The Finest PS1 Emulators for Seamless Gaming Experience
- [Updated] In 2024, Harnessing Hobbies for Hefty Helpings on Digital Domains
- [Updated] In 2024, High-Definition Capture Top Applications
- AI Assistant for Adventurers: 6 Techniques with ChatGPT at the Ready
- Craft a Tailored Communicator: Build a Unique GPT
- Creative and Entertaining iOS Shenanigans to Surprise Your Mates
- From Idea to Output: The Journey of AI-Powered Content Generation
- Get the New Version of Brother Printer Drivers - HL-L2300D Edition
- Harnessing Powerful Conversations with FreedChat
- In 2024, Best Anti Tracker Software For Apple iPhone 12 Pro | Dr.fone
- In 2024, Professionalizing Your YouTube Income with Universal Tech Tips
- Navigating ChatGPT in a Portable World
- Switching to Claude 3? Here's Why It Beats GPT-3
- The Digital Dialogue: Snapchat's MyAI vs OpenAI's GPT
- The Elixir of Immortality | Free Book
- Truth Talks - Busting 9 Common Chatbot Misconceptions Today
- Unleashing ChatGPT's Potential on Mobile Platforms
- Why Does Premiere Pro Keep Failing in Windows 11/10? Expert Tips to Stop the Collapse
- Title: The Language Titans: Dissecting BERT and GPT's Roles
- Author: Brian
- Created at : 2024-11-10 18:18:46
- Updated at : 2024-11-17 19:25:09
- Link: https://tech-savvy.techidaily.com/the-language-titans-dissecting-bert-and-gpts-roles/
- License: This work is licensed under CC BY-NC-SA 4.0.